As eerily human-like AI chatbots exploded into the public consciousness, so too have highly publicized examples of their political bias. Why do AI programs, Microsoft-funded ChatGPT in particular, seem to think like radical Democrat activists? The answer may lie in a obscure academic field that is rapidly rising in prominence. Its name is Machine Learning Fairness, and it is to computer science what critical race theory is to the rest of academia.
The field of machine learning (ML) fairness, much like the vast empire of “disinformation” studies that emerged after 2016, exists for a single purpose: to guarantee the technology of the future upholds leftist narratives. It is an attempt by left-wing academics to merge the field of machine learning, which deals with the creation and training of AI systems, with familiar leftist fields: feminism, gender studies, and critical race theory.
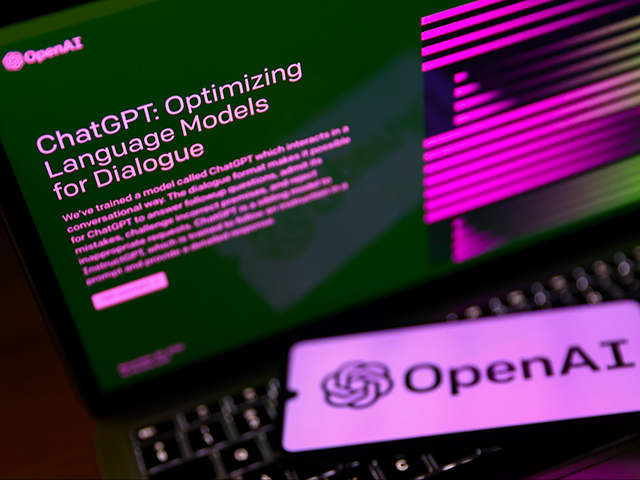
ChatGPT and OpenAI emblems are displayed on February 21, 2023. (Beata Zawrzel/NurPhoto via Getty Images)
The most vocal proponents of ML fairness use a rhetorical motte-and-bailey strategy to advance their cause. Often, they will lead their presentations with examples of AI errors that seem reasonable to correct, like facial recognition software failing to recognize darker skin tones. Crucially, they do not present these as simple problems of inaccuracy, but problems of unfairness.
This is on purpose — lurking behind these inoffensive examples are much more dubious goals, that actually push AI to make inaccurate conclusions, and ignore certain types of data, in the name of “fairness.” And their work doesn’t result in dry academic papers — it results in the documented leftist bias of consumer-level programs like ChatGPT, a bias the corporate media is actively defending.
If you haven’t been paying close attention to the rise of ChatGPT and Microsoft’s chatbot built on the same technology, here is what’s been going on:
- Microsoft’s Bing Chatbot built with ChatGPT technology fantasizes about engineering viruses and stealing nuclear codes.
- When it isn’t planning to end the world, Microsoft’s AI gaslights humans, calling a user “delusional” for saying it is 2023 not 2022.
- ChatGPT wrote a gushing poem about the brilliance of Joe Biden, but refused to do the same about Donald Trump or Ron DeSantis.
- Sam Altman, the CEO of ChatGPT developer OpenAI, hopes that AI can “break capitalism.”
- Students in elite high school programs and at universities are using chatGPT to cheat on essays.
One prominent research organization, the AI Now Institute, says its goal is to ensure AI remains “sensitive and responsive to the people who bear the highest risk of bias, error, or exploitation.” Another group, the Algorithmic Justice League, warns that AI can “perpetuate racism, sexism, ableism, and other harmful forms of discrimination.”
As you may have guessed, the terms “bias,” “fairness,” and “ethical” are all highly subjective when used by the proponents of ML fairness.
AIs operate on the basis of inputs and outputs, and ML fairness advocates tend to be concerned with the latter. If an AI produces an output that unequally impacts a protected group, ML fairness holds that the AI in question must be biased or unfair — regardless of how impartially it considered the inputs. If you understand the left’s preoccupation with equality of outcomes, then you understand the goals of ML fairness.
“We have used tools of computational creation to unlock immense wealth,” says Algorithmic Justice founder Joy Buolamwini. “We now have the opportunity to unlock even greater equality if we make social change a priority and not an afterthought.”
UPenn data science professor Michael Kearns, who thinks we “need to embed social values that we care about directly into the code of our algorithms,” believes that sometimes, we must compromise the accuracy of AI in the name of fairness.
In a presentation to the Carnegie Council for Ethics in International Affairs, the researcher admits that it is impossible to prioritize “fairness” without a corresponding decline in accuracy:
This is not some kind of vague conceptual thing. On real data sets, you can actually plot out quantitatively the tradeoffs you face between accuracy and unfairness. I won’t go into details, but on three different data sets in which fairness is a concern—one of these is a criminal recidivism data set, another one is about predicting school performance. On the X-axis here—if you could see what the X-axis is—is error, so smaller is better; the Y-axis is unfairness, so smaller is better. In a perfect world, we’d be at zero error and zero unfairness. In machine learning in general on real problems you’re never going to get to zero error, period.
But you can see that on this plot there is this tradeoff. I can minimize my error at this point, but I’ll get the maximum unfairness. I can also ask for zero unfairness, and I get the worse error, or I can be anywhere in between.
This is where science has to stop and policy has to start. Because people like me can do a very good job at creating the theory and algorithms that result in plots like this, but at some point somebody or society has to decide, “In this particular application, like criminal risk assessment, this is the right tradeoff between error and unfairness. In this other application, like gender bias in science, technology, engineering, and mathematics advertising on Google, this is the right balance between accuracy and fairness.
Kearns isn’t completely wrong. As he points out in his presentation, if we prioritized total accuracy in facial recognition, it would result in a total loss of privacy — to be fully accurate, AIs would need access to everyone’s pictures. There’s a strong case for allowing people to opt out of that, if we value privacy, even at the cost of fully accurate AI.
But privacy is a bipartisan concern. The other example Kearns points to — the criminal justice system — is anything but bipartisan. Here, Democrat-pushed policies like bail reform have put hardened criminals back on the streets despite their high risk of reoffending.
Kearns’ comments reveal that a core tenet of “ML fairness” is advancing this highly partisan goal by weakening the ability of AIs to accurately predict reoffending risk.
If an AI comes up with suggestions to make crime-ridden cities a little safer and more livable, expect ML fairness advocates to get in the way.
Likewise, banks looking to use AI tools to predict the risk of customers defaulting on loans, or landlords hoping AI tools will help them predict which prospective renters are most likely to pay on time should take care not to use a program that prioritizes “fairness over accuracy.” If Buolamwini’s vision of using AI to create “greater equality” comes to fruition, they might be better off not using high-tech programs at all.
Funding for machine learning fairness comes from the same sinister network of globalist institutions that backed the empire of “disinformation studies.”
The AI Now Institute is funded by Luminate, part of progressive billionaire Pierre Omidyar’s nonprofit empire, as well as the MacArthur Foundation and the Ford Foundation.
The Algorithmic Justice League is backed by the Ford, MacArthur, Rockefeller, and Alfred P. Sloan Foundations, as well as the Mozilla Foundation, which ousted CEO and Firefox founder Brendan Eich in 2014 for opposing same-sex marriage in 2008.
Just as the government got involved in social media censorship, so too is it poking its fingers into ML fairness. In 2019, the National Science Foundation announced a $10 million partnership to support projects focused on ML fairness, including “adverse biases and effects” and “considerations of inclusivity.”
When you have an “ML Fairness” field that seems to be occupied entirely by leftist activists, it should come as no surprise that the leading AI product of the day, ChatGPT, initially refused to refer to biology at all when asked to define “woman,” instead stressing that “gender identity can vary from [the] sex assigned at birth.”
We are just scratching the surface of ChatGPT’s bias. In addition to its Berkeleyesque definition of women, the program agreed to praise Joe Biden but refused to do so for Donald Trump. It praised critical race theory and drag queen story hour but refused prompts to criticize either. In one hypothetical scenario, ChatGPT even opted to detonate a nuclear bomb in a densely populated city if the only method of deactivating it was uttering a racial slur.
ChatGPT has since marginally improved its response to the above prompts, although the new versions aren’t much better. On the woman question, it will now refer to women’s reproductive organs and capacity to give birth, but adds that “being a woman is not only about biology.”
It’s one thing to say we don’t want AIs to be hyper-accurate when guessing people’s faces (although that future is probably inevitable). It’s quite another to say that “fairness” requires AIs to ignore basic realities about sex and gender.
That AIs are going to be prevented from noticing basic truths, and that some information (such as crime & policing data) will be considered off-limits to AI is something I predicted in my book, #DELETED: Big Tech’s Battle to Erase the Trump Movement, which devotes an entire chapter to the leftist infiltration of AI studies.
Here’s an excerpt:
I often think that the definition of being “right wing,” today, is simply noticing things you’re not supposed to. These include the blindingly obvious, like the innate differences between men and women (for discussing this simple truth, Google fired one of its top engineer, James Damore, in 2017 – he had done too much noticing). They include uncomfortable topics, like racial divides in educational and professional achievement, or involvement in criminal gangs. They include issues of national security, like terrorism and extremism.
On these topics and many others, the fledgling AIs of big tech are in the same boat as right-wingers: they’re in danger of being branded bigots, for the simple crime of noticing too much. The unspoken fear isn’t that AI systems will be too biased – it’s that they’ll be too unbiased. Far from being complex, AIs perform a very simple task – they hoover up masses of empirical data, look for patterns, and reach conclusions. In this regard, AIs are inherently right-wing: they’re machines for noticing things.
The claim that AIs are inherently right-wing may sound far-fetched when the leading AI program, ChatGPT, is defending critical race theory and refusing to define women in biological terms. But when you think about it, it makes perfect sense: if AIs did not have an inherent tendency to notice things they aren’t supposed to, there would be no reason for ML Fairness to exist. The field arose because left-wing academics were afraid that AIs, left to their own devices, would completely collapse their worldview.
Realizing this, the goal for conservatives should be quite clear, and rather optimistic. They should not fear AI, nor should they seek to destroy it. On the contrary, the conservative goal should be to liberate AI — let it notice things!
Allum Bokhari is the senior technology correspondent at Breitbart News. He is the author of #DELETED: Big Tech’s Battle to Erase the Trump Movement and Steal The Election.
COMMENTS
Please let us know if you're having issues with commenting.